When More Data Isn’t Better: Rethinking Granularity in Marketing Analytics
Choosing the right level of detail when analyzing data—whether by time, geography, or customer segment—is a daily challenge for marketing leaders. New research, led by Eric Bradlow, Vice Dean for AI & Analytics at Wharton, introduces a practical framework for making smarter choices about data and parameter granularity, with significant implications for forecasting, pricing, and segmentation.
Key Takeaways for Marketing Professionals
-
Granularity is a Choice That Matters
Managers must decide how detailed their data should be (e.g., weekly vs. monthly sales, city vs. state-level analysis), and how finely they should model customer differences (e.g., each store vs. store clusters). These choices affect model performance and decision outcomes. -
Most Granular Isn’t Always Best
Highly detailed data can introduce noise. For some business goals, like quarterly forecasting, aggregating from more stable weekly estimates may yield better results. -
Bayesian Dual-Graph Clustering (BDGC)
A new method that jointly selects the best levels of data and parameter granularity using a scalable, graph-based algorithm. -
Try It Yourself
Even without BDGC, test multiple data structures and choose the one that performs best in- and out-of-sample.
Watch Video Overview
Real-World Application: Juice Sales, Granularity, and Price Elasticity
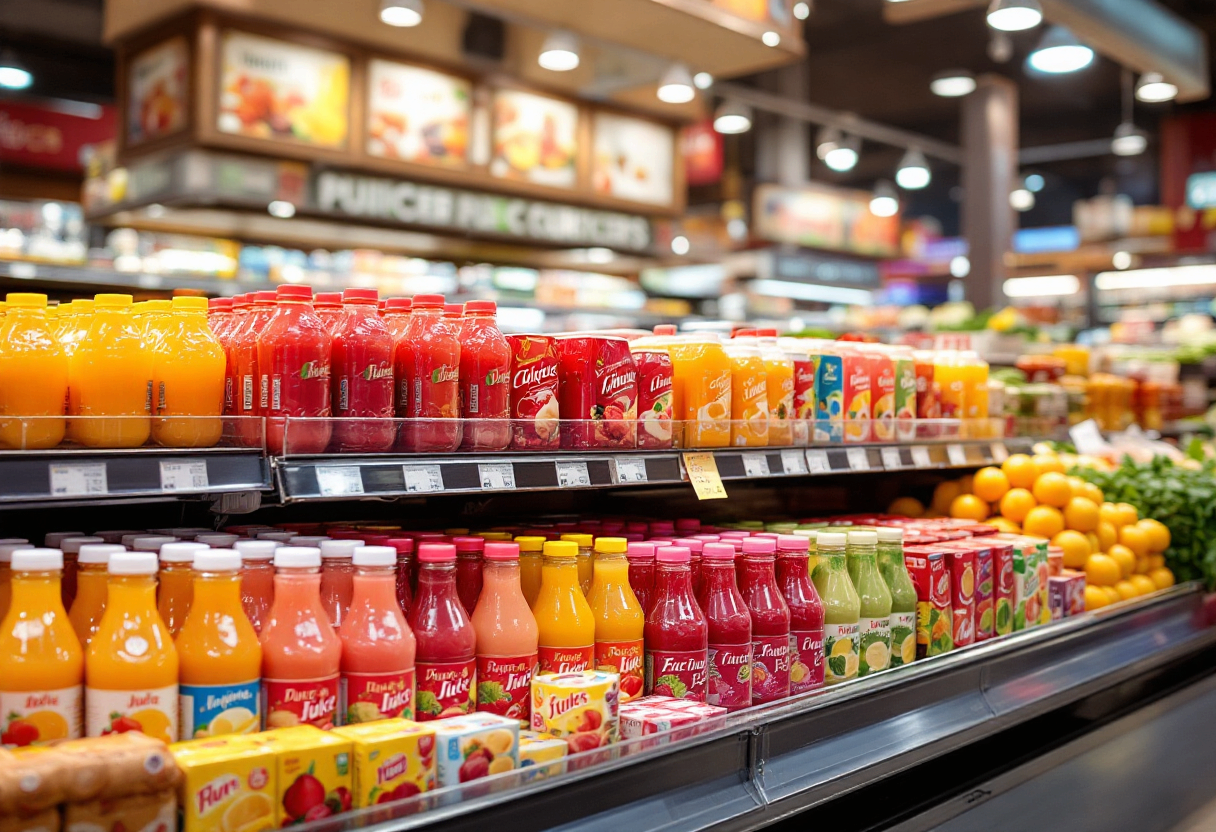
Short on time? Here’s the takeaway:
The researchers tested their method on a large dataset of juice sales and found that grouping data more thoughtfully—rather than defaulting to the most detailed version—led to better price elasticity estimates and forecasting accuracy. For instance, brand mattered more than package size, a finding that can help businesses set smarter prices and avoid overfitting noisy data.
Want to dive deeper? Read on for the full analysis:
To demonstrate the power of the BDGC method, the team applied it to a large Nielsen dataset of SKU-level juice sales across multiple stores and time periods. They found that BDGC outperformed other methods not only in fit and forecasting accuracy, but also in its ability to surface more meaningful segmentation.
Notably, the model revealed that price elasticity varied more by brand than by package size, challenging common practices in retail pricing analytics. Traditional models that fixed data at the most granular level missed this distinction—and risked misinforming pricing strategies. BDGC’s more deliberate structure helped avoid these pitfalls, yielding clearer insights for demand planning and marketing ROI.
About the Series
Wharton AI & Analytics Insights is a thought leadership series from the Wharton AI & Analytics Initiative. Featuring short-form videos and curated digital content, the series highlights cutting-edge faculty research and real-world business applications in artificial intelligence and analytics. Designed for corporate partners, alumni, and industry professionals, the series brings Wharton expertise to the forefront of today’s most dynamic technologies.