Using Data to Engage, Retain, and Grow Banking Customers
OVERVIEW
Fulton Bank is an American regional community bank, based out of Lancaster, Pennsylvania. They serve both consumer and business customers. Last year, Fulton Bank partnered with AI and Analytics for Business (AIAB) and participated in our annual datathon in order to solve business problems and facilitate a stronger adoption of data analytics within their company.
OBJECTIVE
Working with teams of Wharton students, Fulton Bank established five bespoke business units for the datathon, each focusing on how to best use data analytics to answer a pressing business question. The teams were: consumer, commercial, finance, human resources, and operations. Certain projects and other information have been omitted for the purposes of privacy, but the following case study illustrates the value that the Fulton Bank datathon was able to provide.
The Wharton datathon experience emphasizes the power of bringing diverse people with various experiences to focus on solving a business problem. I loved the energy, innovative thoughts, professionalism and drive each team brought to the table. The students engaged brought fresh new perspectives to looking at our data and came up with new recommendations. I look forward to our second datathon!
Jad Abou-Maarouf
Chief Data Officer
Fulton Financial Corporation
Commercial
What product(s) should the Fulton Bank sales team prioritize with a given client?
The commercial business team sought to understand how they could best cross/up-sell certain product offerings. In reviewing Fulton Bank’s data, the team quickly realized that the top five most-popular new products were not consistent across customer segmentation and region. Using logistic regression from client product choice data, the team was able to uncover vital factors of purchasing decisions, and subsequently rank every product/client combination.
Through market basket analysis and other methodologies, the commercial team established subsections of customers based on region and segment, and identified products that were statistically significant indicators of purchasing behavior. The team learned that customers that already had certain products were 16 times more likely to cross-buy other offerings.
Finance
How can we attract and retain more customers from the Millennial generation?
To increase Fulton Bank’s appeal to the Millennial population, the Finance team examined common traits among Millennial customers, and investigated how to better fit their needs. Initial findings revealed that Millennial funds, as they increase, remained in low interest-bearing accounts, and less than 1% of Millennial customers had a brokerage relationship with Fulton Bank.
The finance team recommended that Fulton Bank offer Millennials a bundled checking and brokerage account, providing them with a digital solution to assist them in the management of their money. They also suggested offering an enhanced banking experience, one that incorporates a more robust budgeting/analysis tool allowing Millennials to set and track financial goals.
Consumer
Which consumer households are most at risk of churn (leaving the bank)?
Using a consumer dataset provided by Fulton Bank, this team of students first defined the company’s customer segmentation using a series of machine learning methods – namely Principal Component Analysis, unsupervised learning, and k-means clustering.
The result of their work presented the students with five well-defined customer segments: Swing By, Active, Valuable, Techy, and Loyal. The churn rate of these segments is informed by unique circumstances, with Swing By customers (lowest average mobile logins, highest percentage of closed accounts) having the most churn, and Loyal (highest average age, largest percentage of consumers with high income) customers having the least churn.
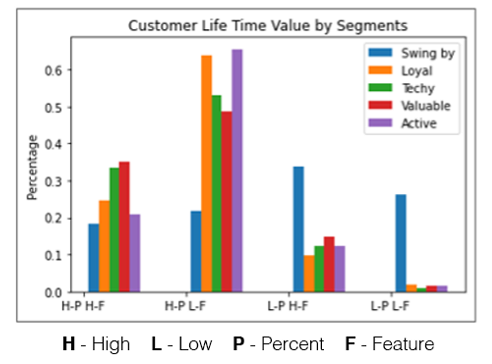
The consumer team provided Fulton Bank with a comprehensive understanding of their customer segmentation, and actionable insights for targeted reductions in churn.
Operations
The Fulton Bank operations team wanted to achieve four main goals by participating in the datathon:
- Determine the relationship between transactions and per item costs (fixed, variable, one time)
- Facilitate business financial planning by forecasting future expenses based on volume
- Utilize data intelligence to strategically renegotiate service provider cost structure and discounts
- Price services optimally for customers
The team identified Fulton Bank’s total cost per transaction and determined what portion of that is driven by volume versus minimum monthly expenses. The team also explored various potential insights relating to the ACH customer usage and experience, based on geography, Fulton Bank’s markets, and aged-based generational demographics.
The operations team succeeded in developing a scalable model for estimating the number of transactions and the corresponding costs of an acquisition when acquiring new branches.
Thanks to the datathon, Fulton Bank has gained a deeper understanding of their customers and the transactions they make, helping them to align their per-item costs more optimally with their product set.