Research Spotlight
Using Machine Learning to Distribute Aid in Sierra Leone with Angel Chung
In Wharton AI & Analytics Initiative’s Research Spotlight series, we highlight research by Wharton and Penn faculty, doctoral students, and researchers whose work focuses on innovations and applications of data science, analytics, and artificial intelligence.
This month, we spoke with Angel (Tsai-Hsuan) Chung, a second-year PhD student in Wharton’s Operations, Information and Decisions (OID) Department, about her work in Sierra Leone on optimizing health supply chains with decision-aware machine learning.
What problem is your work addressing?
In 2010, Sierra Leone launched the Free Healthcare Initiative (FHCI) to provide free healthcare service and medicines for pregnant women and children under five years of age. The National Medical Supplies Agency (NMSA), a part of Sierra Leone’s Ministry of Health and Sanitation (MoHS), was established to ensure the transparent, cost-effective, and timely availability of medical supplies and free healthcare products to every public health facility in the country.
The NMSA faced a number of challenges in the distribution of these services, such as:
- 70-80% of the essential medicine supplies Sierra Leone receives comes from international donors. This presents the challenge of limited and highly variable inventory from which the NMSA can work.
- Health facilities in underserved areas are sometimes understaffed, resulting in missing or incomplete data entry and subsequent data equity concerns. Any predictions or decisions based on the available data may fail to reflect the actual needs of these vulnerable populations.
- Disparities and inefficiencies in the health supply chains compromise well-being and may even facilitate the illicit trade of medicines between government hospitals, drug importers, and retailers.
Due to these challenges, 40% of patients were being turned away without receiving the medicines they needed. As of 2019, only 39% of the country had achieved universal health coverage in Sierra Leone.
Angel Chung
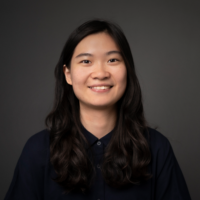
Angel (Tsai-Hsuan) Chung is a second-year PhD student in Wharton’s Operations, Information and Decisions (OID) Department. She works with Hamsa Bastani, Associate Professor of OID and Faculty Co-Lead of the Wharton Healthcare Analytics Lab, and Osbert Bastani, Assistant Professor in Computer and Information Systems with Penn Engineering. Her research focuses on developing data-driven decision-making tools using machine learning and optimization for social good, education, and equitable healthcare and global health operations.
What methods have you used to address this issue, and why?
A reasonable machine learning approach would be to forecast demand for various health products at each health facility and then optimize the allocation of a limited supply of health products to minimize unmet demand across health facilities. We build on this approach, introducing several methodological innovations to improve efficiency and equity.
Traditional machine learning focuses solely on prediction accuracy but overlooks our ultimate goal of allocating limited resources effectively to reduce unmet demand. For instance, the prediction accuracy for a health facility with ample stock isn’t as critical to us; we would prefer to prioritize higher predictions for facilities facing shortages. Therefore, we developed a decision-aware learning framework that can identify and focus on the predictions and allocations for health facilities more likely to experience low supplies, ensuring people have access to the medicines they need.
Also, to tackle the issue of data equity, we utilize more comprehensive, alternative data sources – collected from the Sierra Leone census, satellite data, and multilateral organizations like the United Nations – that enable us to estimate the demand from the at-risk populations served by each facility. We incorporate this into our machine learning model to enhance efficiency while promoting equity.
Finally, we have been working closely with local frontline workers and government officials at the NMSA and the MoHS to tailor our model to the real needs on the ground. I have visited Sierra Leone twice to understand the nuances of the local government policies and operations. We have deployed our framework for the allocation of essential medicines across all government-owned healthcare facilities in the country. To ensure sustainable impact of our approach, we have also developed a web application with an intuitive user interface, allowing Sierra Leone government officials and staff to continue making use of our framework.
What impact does your solution have?
We used a synthetic difference-in-differences model to evaluate the impact of our approach. Our result shows around 20% improvement in people’s access to essential medicines and medical supplies by the second quarter of 2023. While introducing an innovative change into an existing government system is tremendously difficult, we have successfully incorporated AI technology on a national scale and showcased improvement in this resource-constrained setting. Our success demonstrates not only the effectiveness of machine learning for resource allocation in developing countries but also the transformative impact of research in real-world practice.
What would help improve your solution?
As mentioned above, one of the key challenges we face is the lack of quality data. With more investment in developing a better digital infrastructure for data collection and in cultivating local skilled human resources, we will be able to further improve our model. This will also allow us to expand our efforts to other medical resource allocations, such as vaccines and malaria products, thereby scaling up our tangible impact on people’s lives.
Where can we go to learn more/keep up with your work?
If you want to learn more or keep up with our work, please visit any of the following:
- Angel’s website
- Angel’s X: @AngelTHChung
- Hamsa’s website
- Hamsa’s X: @hamsabastani