Analytics at Wharton
Research Spotlight
Estimating the Effect of YouTube Recommendations with Homa Hosseinmardi
In Analytics at Wharton’s Research Spotlight series, we highlight research by Wharton and Penn faculty, doctoral students, and researchers whose work focuses on innovations and applications of data science, analytics, and artificial intelligence.
This month, we spoke with Homa Hosseinmardi, a research scientist at the University of Pennsylvania’s Computational Social Science Lab, about her recent study, “Causally Estimating the Effect of YouTube’s Recommender System Using Counterfactual Bots.”
What problem is your work addressing?
Whenever problematic behaviors are observed on online platforms like YouTube (such as radicalization), it is generally hard to determine why they’re taking place. Are users or society influencing these behaviors? Is it the algorithm? Or is it a combination of all of these factors? It is extremely difficult to disentangle algorithmic influence from user intentions from what we observe happening on platforms in the real world.
What methods have you used to address this issue, and why?
In my recent study of radicalization on YouTube, I developed a new methodology that combines observation data with designed experiments to disentangle user preference from the recommendation algorithm, making it feasible to causally infer the role of the recommendation algorithm.
Essentially, to help solve this issue, we need to examine how humans might behave in the absence of algorithmic influence. This requires an environment that could only be facilitated by these platforms themselves, so, alternatively, we have to examine what humans’ experience on these platforms would look like if they had no preference or agency themselves. To that end, we have developed what we call “counterfactual bots” to causally estimate the role and influence of algorithmic recommendations on highly partisan content on YouTube. We compared the behavior of bots that replicate real users’ consumption patterns against our “counterfactual” bots, which follow rule-based trajectories, and observed the difference as the contribution of users’ preferences.
Homa Hosseinmardi
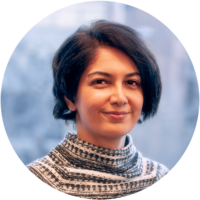
Homa Hosseinmardi is a research scientist at the University of Pennsylvania’s Computational Social Science Lab, led by Duncan Watts. Her research centers around holistic and scaled studies of sociotechnical systems, information ecosystems, and online safety, and she is the lead researcher of the Penn Media Accountability Project (PennMAP).
What have you found?
My study of YouTube indicates little evidence for the popular claim that YouTube drives users to consume more radical political content, either left-wing or right-wing. Instead, to help answer the question of what role these platforms play in the radicalization of users, YouTube, like other social platforms, should otherwise be viewed as part of a larger information ecosystem in which conspiracy theories, misinformation, and hyperpartisan content are widely available, easily discovered, and actively sought out. My study was cited in Congress and also generated a lot of attention in the public and research communities regarding the role we play in the outcome of the algorithms that we use.
What would help improve your solution?
My studies have been limited by desktop usage of sociotechnical systems, and we cannot blindly generalize these findings to mobile, for example. Yet, getting real user trajectories from mobile devices is its own new world of challenges, and expanding my proposed methodology to work on mobile is not a straightforward task.
Where can we go to keep up with your work?
If you’d like to learn more and keep up with my work, please visit my GitHub page.